Poole Podcast Episode 11: Big Data Analytics with Home Depot CFO Richard McPhail, VP Erik Holbrook and Professor Bill Rand
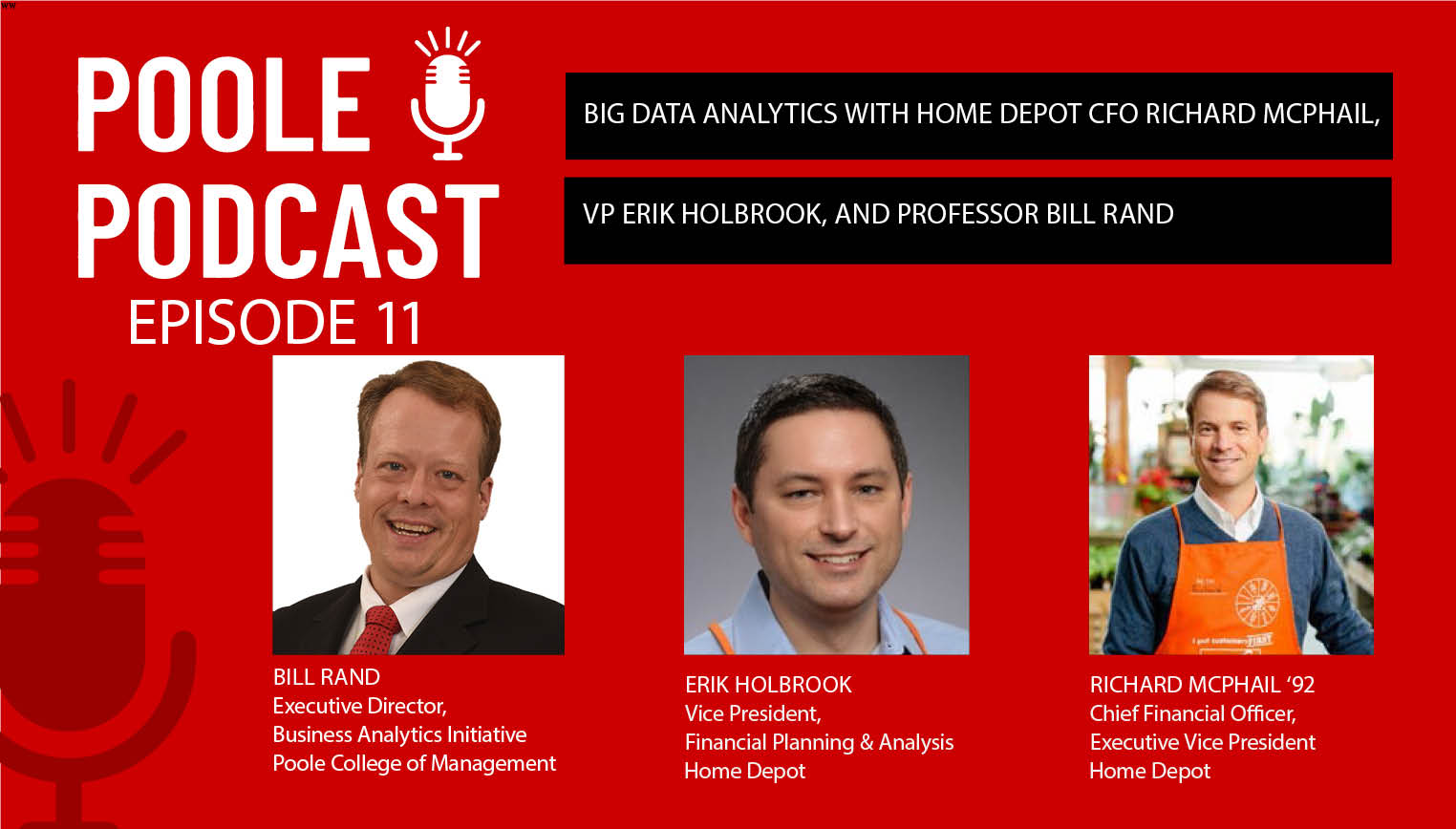
With about 500,000 employees and over 2,000 stores, at The Home Depot, data science and analytics are critically important to solving business problems. But leveraging data and analytics are not just for huge companies anymore. Our guests talk about how every tactical decision should be made with analytics backing it up.
Episode Transcription
Jenny: Well, Bill, let’s start with you. As I mentioned in your intro, among your many roles and responsibilities, one of them is the executive director of the new Business Analytics Initiative, BAI for short. For those that are not familiar with this, do you mind providing us a little bit of a 30,000-foot view of what it is and how important it is to have it in Poole College?
Bill: Yeah. Sure. So, the BAI, it really capitalizes on a lot of investments that Poole College has made in the past. In terms of developing analytics resources. And we’re bringing those all together under one organization called the Business Analytics Initiative that allows us to really excel in that space and provide a new set of opportunities and initiatives in that space as well.
So we really focus on three kinds of areas, teaching of business analytics, right. And at that we have an undergraduate program in business analytics. We also have a graduate certificate in business analyst that compliments nicely with the MBA. And we have a masters of management and marketing analytics that’s going to be launching in the fall of 2021 and we hope to do more of those in the near future. We also look at research, so we employ, we do sponsored contracts where we’re kind of working with companies, organizations, and the federal government to really understand how business analytics can advance the state of the art for decision-making and business processes in the future.
And finally, we hope to play a role in thought leadership, right? Really taking the teaching and the research efforts that we’re doing and spinning them back around to other businesses and other companies can really take advantage of them and see how to best apply business analytics to their own organizations.
Jenny: And Bill, for those that may not be familiar with kind of the culture at NC State, we have a strong data science area here at NC State. How will these units work together if they, they will, or they won’t? What, how does that?
Bill: Yeah, very much. I’m happy that I can now say it publicly. I’ve known about this for a while, but in June of this year, NC State is going to be launching the Data Science Academy and that’s been announced, right? And the Data Science Academy is going to be bringing together all the different data science efforts at NC State University.
The BAI will serve as Poole College’s bridge, if you will, to those efforts, right? So we’ll be working with computer science, we’ll be working with statistics, we’ll be working with other disciplines, right, that med whoever, right. To kind of try and really take advantage of all the great data and engineering opportunities that we have in the university and applying them as much as we can to help businesses, organizations solve problems and what BAI will be doing is bringing kind of the business acumen and the business knowledge to that space, right. So we’ll be presenting how we can best help that to make a better business decision.
Jenny: Awesome. Richard, we’re going to dig a little bit deeper about your involvement, Home Depot’s involvement and kind of data science, but I wanted to start by saying, I of course read your bio. And, you did your undergrad here at NC State and Poole.
Richard: Yes.
Jenny: We won’t mention where you did your masters, kind of a dark blue school down the road, but we’ll, we won’t hold that against you.
But, I’m curious, you know, how did you decide finance? What drew you to this industry and kind of this space?
Richard: Well, I was always interested in business. I was also kind of quantitatively oriented, and so I think for me, NC State really lit a spark in my mind and kind of showed me that deep quantitative skills actually give you an advantage in business and finance. And you know, I actually, I need to call out a professor with whom I haven’t spoken probably in decades, but Paul Fackler, who I think is still in the department of economics had a just an awesome futures and options course that was deeply quantitative. I think it taught me for the first time kind of the power of quant in applied economics or applied finance.
And so, you know, I, I want to give him credit for opening my eyes, but business is just a form by which we create value for a customer, and we create value for a shareholder, and in the middle, there is kind of the art and science of understanding how to respond to customer demand, which is a big part of, you know, of, of data science and what we’ve done.
But I love my time at NC State. Go pack. Yes, I did go down the road. but I was a Wolf Packer, born and bred, so good to be on the podcast
Jenny: Thank you. We’re so glad to have you. And you brought along with you, Erik Holbrook, who clearly is kind of the mastermind behind the Data Science Forum at Home Depot. Erik, maybe you could talk to us a little bit more about that, why it was created for the Home Depot organization, and tell us a little bit more about how it helps support the strategy of Home Depot as a whole.
Erik: Thank you, Jenny. And again, thank you for having me today. so when you think about data science at Home Depot, and you think about the data science forum, I guess just stepping back, when you think about how companies organize around analytics, there’s a number of different ways to think about that.
At the two extremes, you have kind of a decentralized model and then a centralized model and at Home Depot, we are, have been focused on kind of being decentralized and having those, the analytics teams as close to the business problems as they can be, but the data science forum is really the bridge from that de-centralized model to the benefits that you get in the centralized model.
And so when you think about what we do with the forum, we’re focused on, you know, driving an amazing associate experience for our data scientists. We want to, to hire the best associates and give them a consistent career path and development opportunities across the organization. And so, you know, we talk, talk about NC State and unfortunately, I didn’t go to NC State, but I’ve spent a number of you know, made a number of trips to NC State over the years, recruiting NC State graduates, many of whom have developed just wonderful careers at Home Depot, working in analytics and data science.
The second thing that, that the forum is focusing on is technology and all the tools, and then we might talk a little bit more about that. And then the, the last one is, as you know, as you said, Jenny, kind of, how do we leverage data science and analysts, to help make decisions at the company? And so there, I mean, we have teams across the organization focused on things like how do we improve our online shopping experience?
How do you think about, you know, optimizing our supply chain, how do we deepen our relationships with our customers and you know, how do we get the most productivity out of bays in our stores? And so again, all of those teams are working hand in hand with business partners to look at those problems.
And, as Richard said earlier, ultimately the goal of all of this is to enhance the customer experience or the associate experience at Home Depot.
Jenny: And tagging along on that, Bill, I would say you work with a lot of different companies as clients in kind of in a consulting type of basis, what would you say, what are you seeing companies do and how are they leveraging data and some of their strategy?
Bill: Yeah. I mean, I think one of the things that companies are doing well, especially the more powerful companies, the larger companies I should say, right, is it starting to in-house their data analysis and their data science efforts, right? There was a culture for a while that you would basically give your data up to other organizations who would then process it and provide you insights back.
Right? Whether they be consultants or whatever. You know, I think that that mindset is starting to shift right, in many respects, and I think something like the data science forum and Home Depot kind of are an example of that, right, that you really need to start looking at the data yourself.
People often say data’s the new oil but that’s not quite true. Right, because data can be reused many, many times where oil can only be used once. And if you’re kind of giving away your data and allowing someone else to run through the analysis and looking at it and then providing you insights you’re essentially giving up a wealth of actionable insights that you could generate down further down the line from that data.
Right and so I think that it’s very important for firms when they have the capabilities and when they have the resources to process and analyze that data themselves, right. Besides no one else knows the context of your business as well as you do and so who else has better operators better, skilled, or better prepared to deal with and analyze that data than you are yourself?
Now one problem that a lot of companies face in this regard is they simply lack the internal human resources to really analyze and examine data at large scale. And that’s one thing we’re hoping to solve in the BAI, right? Among others, right, is to try and prepare students and all the different career paths to really be able to start to make data-driven decisions, no matter where they are, no matter where they sit in the organization.
Jenny: And that’s a great lead into this next question for you, Richard you’ve had several roles over your career in finance, and in your role now as a CFO, I imagine, or maybe don’t imagine that how analytics plays in so many of the decisions that you make for the company. Do you anticipate that that is something that will continue here in, in, in the CFO or it just, the C-suite in general moving forward and how much has it changed the role of finance per se and an organization?
Richard: Well, I think we’ve been on an evolutionary path where finance professionals and even just business generalists have seen part of their job at being pattern recognition. And so the example I would give is, you know, even I’d call it three years ago, there would be folks who would probably measure their self-worth by the thickness of the binder and the number of the reports that were in that binder on a Monday morning.
And so your ability to digest information, you know, if at all possible, recognize some patterns in the data, was kind of the key to self-worth and to work in the organization. You know, just as a reminder, Home Depot reported sales of $132 billion last year. you know, we have over 2 billion or close to 2 billion customer transactions every year.
And so thinking about pattern recognition at that scale is impossible. What I think we’ve evolved into is trying to allow humans to do what only humans can do, which is interpret signals, interpret trends that are not detectable by a human because there’s just too much there. And then making that next step to action. So I think that the role of data science for me as a CFO is, I don’t want to do analysis anymore, you know, I can do it, but there’s so much work in separating signal from noise that I would bury myself.
I’d say that my impact on the organization is taking what our data scientists see us signal and interpreting it and making decisions to orient the company in the right way, you know, and an example of this, really, and Erik built all this, by the way, just to hats off to Erik. And he has kind of a unique background that I’ll get into in a second, cause I think it’s critical for leadership and data science. We realized that we had this problem with pattern recognition and so we created this concept of. data science identifying trends and really kind of raising up the headline. And you know, that that’s kind of the first step, I think, in what data science does for finance. Where’s the transparency, what is signal what’s noise?
The second step is when you’re getting into cause and effect. Okay, if that is signal versus noise, then why did A happened? Did A happen because of B or because of C? You can’t do that with deep, without deep statistical chops. And I, I kind of, co-mingle all of that into the world of quantitative business analytics that maybe you call it a science or not, but we can get into the evolution of Erik’s role in a minute. But that that’s, that’s how I see my world changing.
Jenny: You mentioned this Richard, there’s just so much information. And so, you know, Erik in your role, and I guess kind of just in general, how do we get past the data overload and to the point where it doesn’t consume us? And I see oftentimes companies, they get stuck, right.
They can’t see past that. They can’t take, as Richard said, the pieces that they need and move on. How do you anticipate that? And do you see that often in other organizations as well?
Erik: Yeah. I mean, Richard, Richard alluded to this, I mean, you think about Home Depot and, you know, you know, almost 2 billion transactions in a year, you know, there is a lot of data and a lot of organizations have a lot of data, and I think as you think about where you get stuck, I mean, I think, and this is going to sound very simple.
But I think you, you get stuck when you lose sight of what you’re trying to do and the business questions you’re trying to answer. And when you get so enamored with getting to the, the perfect data set, instead of getting to an answer using the data I think is, is something that that I’ve seen happen that, you know, it can be a struggle.
Because again, getting at the information in some cases is a lot of the work and a lot of the, the efforts that go into developing the analytics and, and getting to the answers. But at the same time, I mean, I think, you know, kind of talking about Richard’s example a little bit more, we are in a place where more than any time before we have all of this information and we have absolutely outstripped kind of, you know a human, you know, human ability to kind of pour through everything that we have.
And we’ve solved a lot of the problems you can solve with the information that you had in the binder right, and so we’re at a point where you’re saying, hey, we need to go deeper into this and we need to kind of get to that next, that next set of problems. And that’s where you think about, artificial intelligence and machine learning and all of these advanced techniques that require so much data to get to the answer.
And how do you kind of leverage those techniques? How do you get into the information more deeply, to really get to the place where, you know, you’re pulling from the data, the information that is the most important, you know, try and figure out how to make that actionable for somebody and make it digestible? And then, you know, again, taking the feedback you’re getting from that, and how do you put that back into the, to the feedback loop to continue to train the models?
It is hard to get all of the data ready. You have to stay focused on the question you’re trying to answer. You have to iterate you have to have tools and technology that allow you to, to learn and change kind of constantly as you’re doing this work. I think those are some of the keys to being successful.
Jenny: Bill tagging along on that, Erik mentioned the AI and how that’s playing a role kind of in this analytics space, where are you seeing this implemented? And if you’ve seen some great examples of how companies are leveraging AI now?
Bill: Yeah, for sure. And I think a lot of what I’m going to say here is going to resonate a lot with what Erik and Richard just said, right. You know, really the role of AI is in trying to take away some of the things that humans used to do that they weren’t very good at and basically doing that. And so, you know, computers aren’t yet at the level where they are doing as well as humans on every single task and every single way, they’re not replacing us, in any sense of the word anytime soon, right.
But there are certain things that computers do better than we do, right. And that’s really where AI has its biggest bang for the buck, right, is in doing those kinds of tasks, right. I like to think of AI and analytics as a whole, I like to think of it as proceeding through a couple of stages, right. We had descriptive analytics, which was essentially business intelligence, right. That was like telling you where your company is right now. What the, what it looks like at this point, you don’t really need AI for that.
That’s really just counting right, to a large extent really, but then you move on from that to predictive and yeah. Right, which is being able to tell you where the company is going in the near future, right, and that’s starting to get into AI. You know, some of it can be done with kind of straight up statistical regression methods that, you know, don’t really involve modern what we consider to be AI methods.
But then finally you get into prescriptive methods, right. Which is telling you prescriptive analytics, which is, what should my company be doing in the future? Not just where is it going, but what should they do? Right. And that’s where I think AI really kind of plays a larger role.
Richard: And I think we can actually bring that to life. It is a great way to structure how you think about it. So first stage is where do you stand? And we, we started there. And by the way, incredibly critical function. Then the predictive. I’ll give you an example. There so example, the first one is what’s happening in sales, right?
Uh, am I getting signals that are telling me there is a problem for us in, in paint, in the southwestern US, or is this a demand problem or is this an inventory problem? Right? So where do we stand? Maybe there’s something we do about it.
The second level predictive, give an example from last year. So when COVID hit, it’s kind of the middle of our first quarter last year, and the country kind of went into lockdown, we went deeply negative in terms of sales versus last year. Then, within a period of days, once the fed and the treasury had acted and the government enacted, we sprung back to double digit positive growth year ever, which for us is unprecedented in our size. You think about what that means for us. Well, when you have unprecedented demands, you have unprecedented flow of products onto your shelves and off of yourselves.
And so the applied problem is how do we stay ahead of demand in an unprecedented environment? How can we make sure we are actually ordering the right amount and the right mix of product to keep up with patterns that we’ve never even seen? And so the good news is we have a deep developed competency in data science in our supply chain, and it’s because of that deep competence that we were able to stay in stock for our customers and be there for them when they needed us.
The third level you know, I might describe it a little bit differently and it would be kind of active, Transformation of your business model and, we might all interpret that differently, but mine would be like, you know, homedepot.com which represented around 14% of our sales in 2021 of the largest e-commerce platforms in the country is, in essence, in the business of making sure that we attract traffic, but we also convert that traffic. And the more we know about you, the more able we are to attract you and convert you. Well, it takes a lot to know who you are. It turns out it takes data science to pull out trends and apply them to what we already know about you.
You know, your behavior on the website, your behavior with us, your purchasing behavior, and then say, all right, here is how we serve up a digital experience to that customer that’s more relevant for them, at that moment, you know, if you’re a professional buying a hundred water heaters a year, we know who you are. We’re going to give you a very different experience on the website then if it’s your first time ever buying a water heater and data science powers, all of that.
Bill: Yeah, that’d be that’s exactly, Richard, that’s a great example. Actually, I think of, I think we’re on the same page on this, the state, the third stage, which is prescriptive analytics, right? It’s for, in my mind, which is, what is the right offer to offer the right customer at the right time point in order to maximize the customer relationship.
Right. And I don’t mean maximize the customer transaction value, I mean, maximize the customer relationship in the long term, right. so what do you do to make sure that they keep coming back to Home Depot. And you know, kind of as a follow-up since you brought it up you know, a question I kind of have for Richard and Erik.
You know, when the pandemic hit, right. It did shift a lot of the data patterns that we were observing. Right, and a lot of the models that we had built, whether they be in, you know, data science built or not, were kind of trained and embedded in a kind of pre pandemic world. So how was the process that you went going about updating or transforming or changing those models in different ways to address the pandemic and to address any dramatic change in the economy, right? To some extent.
Erik: Yeah, Bill. It’s a great question. And you know, as Richard said, as you said, Bill, I mean, it absolutely was a, is a huge shift and, and all happened very quickly. and I’d say, you know, there are a number of impacts, Richard mentioned our supply chain and our, our online teams.
Um, I mean, all of our teams really that are engaged in kind of looking forward and forecasting for the business. You know, really in a very short amount of time had to understand what the new trend was and make adjustments to their models. And again, we’re very fortunate to have a group of world-class data scientists embedded with all of those teams and helping to do that work.
And you know, the agility and the, and the ability. with the technology that we have to really kind of quickly identify and adjust to those trends. You know, starting in the, in the, you know, kind of the middle of Q1 last year and then, and then really continuing throughout the year. And you know, I’d say it’s.
It’s the associates and their expertise and, and the technology working together. And then also just, again, back to the, some of the comments that we discussed earlier, having the information and the insights, being able to get into the, to the data and use it.
Jenny: Do you feel there are lessons learned that now you have lived through this that you will certainly apply moving forward? or are there things that you’ve learned maybe about your process that you realize you don’t really need anymore? having gone through the pandemic.
Richard: You know, for me, I think it gave us the realization that we are operating on a much shorter cycle than we ever realized. And just maybe to kind of bring that for life to life, for finance people, you know, for, for decades, maybe a century we’ve been oriented around an annual planning process and, you know, setting out what we think the year should look like. What we learned in COVID was no one week told us all that much about what the next week was going to look like.
When the rubber meets the road, it’s actually all about making sure that we have inventory on our shelves and the right number of associates to support our customers and those things can’t be planning on an annual basis, they’re planned as you’re making purchases, as you’re writing schedules and, you know, data science has supported both of those functions. You run the business with a long-term view and a short-term view
The short-term view, I believe is becoming more and more supported by analytics because they’re, you know, its fast insight, it’s speed to action. And so it, it frees me up. And what I, what I learned from COVID was, let those capabilities free you up so that you can think about the long term
Jenny: Erik, any responses to that?
Erik: No. I mean, I absolutely agree with all of that. I mean, I think the one thing I’d maybe add just, just through the analytical lens, is it helped us understand, you know, kind of do the short cycle point, helped us understand kind of how, you know, how to adjust and move more quickly, be more agile.
It helped us understand, you know, a little bit more about, you know, models and how resilient they need to be. And then I think the last thing is, you know, I mean, again, just helped us, you know, just really crystallized priorities and how to think about, taking care of our, of our stores, our associates and our customer.
Jenny: So Bill, I want to pivot the conversation a little bit, cause I think it’s important to talk about this, really preparing our students for the skills that they need or for the jobs we don’t even know exist yet, right. So in your opinion, as, as the director of the BAI and certainly a faculty member, what are you seeing as some of the skillsets that are critical for our graduates to make sure they have, as they step out into the work.
Bill: So first of all, I think more and more analytical and data-driven decision making is becoming important for every career, right? Historically we thought about this maybe in the information systems that maybe an accounting and finance, right. But even in human resources, right. Even in, you know, management, even in all these strategies, all these other marketing, especially in my own field, right.
Having the ability to make data-driven decisions is becoming critically important, right. You know, Edwards Deming, the great management consultants and, and guru kind of said it best when he said in God, we trust. All others bring data, right. You know, it’s becoming expected that if you’re going to present your strategy, your vision for how the company should be moving in, whatever direction, even the tactical decision, right. About who you should hire over someone else, right. That you present data to back that up, right? That you present some sort of explanation as to why that is correct based upon patterns that we’ve observed in the past. Right.
I think part of that skillset, that’s important for the students to have the skills for them to have is model thinking. Right. Which is the idea that almost every decision can be built upon a model, right. That you can sketch out what would go into it. What would be kind of the inputs to the decision? What’s the output you’re trying to achieve and kind of how the inputs relates to the output in some way, right? And even the most abstract decisions can start to be modeled in this way.
And as we get more sophisticated tools, we simply refine the ability to make that decision. Right. We make it better and better over time, right. Erik kind of mentioned this that companies have tons of data out there. Right. They have more data they can possibly process. And a lot of it though is in an unusable format right. People need to be able to figure out ways to transform that data into a set of data that’s actually useful, that can actually help you make a decision, right. A lot of textual data, right. That doesn’t necessarily have an equally easy way to turn it into numbers. Right. that you can use image-based data, right that doesn’t have, that has information contained within it and even video data, right. And, you know, kind of alluding back to some of the points earlier there, right. That’s one of the places AI can really help, right. Is in transforming that data.
I’m not expecting our graduates to come up with being able to write a convolutional neural net on their own right, or anything like that. But I think they need to be able to understand what those kinds of technologies and what those kinds of tools can do for a business and organization. And they should be able to talk intelligently with the people who put those tools and the practice and who make that data available to them so they can make the decisions that they need to.
Jenny: What are some of the things that you’re looking particularly for your organization skill sets and maybe some of the soft skills too, that we don’t always mention, that are important when looking at someone to hire?
Erik: Yeah, it’s a, it’s a great question. And I think Bill, Bill covered a lot, a lot of great thoughts in what you just said. And so I agree with that. I think one other thing I’d add is, when we think about hiring associates in, into one of our data science teams, I mean, it’s really one of the biggest things we look for is curiosity. And wanting to find associates who, you know, because again, again, chances are they’re, they’ve never worked in retail. They’re not as familiar with, with Home Depot. They have a lot to learn to take, you know, kind of what they’re bringing from school and applying that to working at Home Depot. Right.
And what we’ve found is that curiosity is really something that sets, sets those students apart, as they’re looking for roles, you know, kind of brings them with their expertise they’ve learned at NC State, and allows them to kind of hit the ground and Home Depot and, and, and really ask a lot of questions, and, you know, learn the business and learn how to apply the craft of analytics to business problems at Home Depot.
Richard: I agree with Erik. I think just in terms of what students should not neglect in their education is a level of foundational understanding of business and economics, and it’s for two reasons. Probably most importantly, Erik’s reason, we want our folks to be curious, but what’s really powerful is when they say, look, here’s what you’re missing that’s big rather than this seems like it could be big, and typically those things come out of a really strong data competency coupled with some commercial competency.
And not all that has to be picked up in the classroom, you know, go get a job, you know, that isn’t in data science, you know, in the summers. Just, you know, learn what it’s like to, to be out there interacting in a business because there is a practical level. And I think this is probably the second point, you know, to keep in mind. If you want to evolve in your career, you’re going to have to be able to make insights actionable. , And you’re going to have to be able to deal with people like me who are not data science natives.
And, one of the evolutions of Erik, you know, is, is that Erik has been in, in finance his career at Home Depot and, and had a, an incredibly strong understanding of the commercial side of our business worked in business development for years. And so understanding what’s going to resonate with the folks you’re partnering with in business is really important and it’s a critical link and yeah, that’s probably one of the most elusive skills, you know, can you make this translate? And do you understand why it translates?
Bill: Just want to highlight what Richard’s saying in there really quick, Jenny, which is that, you know, there are, in specifically how pool college could help out with that, right. Which is that there are a lot of great data science programs out there. And if you think about it, right, data science really involved translating data into wisdom. Right. and I, I like to think this pipeline of data, information, knowledge, and wisdom, right. And Richard’s really spelling out that they need to have the right business acumens, right. In order to make wise decisions in this space.
And we have Poole College really concentrate on the upper half of that pipeline, right. About on the, on the translation of information to knowledge, to wisdom. Right. And we try and give our students the skills to know, what is a business problem? What is the problem that the business is facing? Right, and that’s really, and I think Erik mentioned this earlier. That’s really where the question should start, right.
It shouldn’t start with what kinds of cool things can we do with this new tool we have, right. It’s just start with what is the business problem, and the differentiation between something like the master’s management and marketing analytics and a data science program, is that you’re going to get well embedded in what marketing problems are about. Right. And what marketing needs to worry about as opposed to just trying to build models of data for any particular space.
Jenny: I’d be curious to see what, what you guys think about this, that we’ve had a couple podcast episodes where we’ve had we’ve talked about entrepreneurship, but I would think in an organization specifically like Home Depot, interpreneurship is just as critical. And I think when you discover ways to problem solve in a unique way, that becomes part of the plan too. How much of that do you think, you know, students should be doing and thinking about when they come to a job, how they can create opportunities within an organization?
Erik: Yeah. So I, I, I think it’s, it’s critically important and absolutely important at Home Depot. Entrepreneurial spirit is actually one of our core values as an organization, and so for us, and you think about data science and analytics, I mean, again, it’s, it’s all about, you know, how do you understand what the business problem is?
And how are you empowered to solve it with analytics? I mean, and again, that sounds very simple, but at the end of the day, that’s really about, you know, making sure that we’re, we’re driving connections you know, between the teams that are doing the analytics and the, and as Richard said, kind of the commercial leaders in the company and, you know, understanding what the problems are and, and, you know, giving the teams and the data scientists the, you know, the tools and information they need and empowering them to go and solve the problems.
These are not things you can solve from the top down in an organization, right. These are problems that have to be solved, you know, at the, you know, in the front lines of the organization for the associates that are kind of there. And in the, you know, working with our customers working with our vendors, kind of at the, on the front lines of you know what the problems are. And that’s where I think, you know, having an entrepreneurial mindset, a commercial mindset, as Richard said a minute ago, and an analytical mindset and kind of bringing all of those things to bear on a problem is, is really how you, how you become successful. and applying data science in an organization.
Jenny: Now, Erik, I have to ask, you know, what excites you most right now about the data analytics space? What do you think is the future and what, what I know getting up in the morning and go and work at Home Depot you get excited about, but what about analytics? Do you get excited about?
Erik: So I think it’s, I mean, it’s really kind of going back to the, you know, to the phases that Bill was talking about in some of the, some of the examples that Richard shared a few minutes ago. I mean, I really think we’re at a place in in the evolution of analytics and data science, where we’re really at kind of a tipping point on, you know, having the technology at our fingertips to kind of, you know, do more and more of, of analytics and solve more of those phase three problems, if you will, you know, those things, you know, kind of getting to that level had always been elusive because, you know, you didn’t have the technology or you didn’t have the data. And we’re really at a place I think, you know, in the, in the world of analytics where, you know, all of that is now kind of. It is in front of us and we have the opportunity to leverage the technology to really kind of get to, you know, that, that third phase in, in more and more of the work that we do across the organization. So again, just get really excited about that, really excited about the application and, and again, all of it, because, you know, ultimately, we’re excited about, you know, kind of better ways to solve problems, right? And better ways to serve customers and better ways to grow our associates.
Jenny: On that front Richard what’s in the pipeline for Home Depot? What are some of the things that you guys are looking to do or hope to do in the next 12 to 18 months, that that are exciting to you right now?
Richard: I think that, more than ever homedepot.com has become the front door of our store. They either order online or go to the store to, to purchase a product and start a project we’re pushing hard in personalizing, and, and the underpinnings of personalization are data science. And so that’s an area that’s, that’s really exciting for us. You know, one one thing we learned during COVID is that now more than ever the home is more central to folks’ lives than it ever has been. a by-product of that is that they’re doing more projects.
When you get to the level of trying to help someone accomplish a project you need to know more about them and you need to know, you know, intent and ability and willingness. And again, all those things are kind of, they’re marketing problems in some respect. And so again, the backbone of marketing and in our online experience is data science. We look forward to the next few years of pushing deeper to understand our customers better. But right now we’re just concentrating on making, making sure that we have a, a safe environment.
Jenny: I understand that. Bill, I guess a follow-up question to you would be now that we’ve got this business analytics initiative, we’ve got the masters of marketing analytics starting in the fall. What do you see as far as analytics and the role of, you know, educating students to be better prepared in the analytics space? How do you see that panning out here in the next couple years?
Bill: I almost have to answer the question you addressed Erik in order to do it right. Which is like, what makes me excited about the future of analytics. Right. and I think that it’s important to like take a step back and realize 20 years ago, computers couldn’t see right? Like they couldn’t analyze image-based data the way they are doing it now. Right. As much as 10 years ago, they couldn’t really analyze text-based data as well as they are doing it now. Right. There’s, we’ve made huge advances in both those fields. Right.
And so to really think about what the future of business analytics for our students looks like, we need to think about what are the future of those tools, right? What are, where are those tools heading? What are the new insights that they’re going to be able to provide to us, right? and I think that’s really exciting, right. And we need to start, and, you know, we, we, haven’t been teaching how to use images in your decision-making processes, and in courses right. Like that’s not something we do right now. We don’t really look at, you know, text on a regular basis, right.
We need to include more and more of that into our coursework. Right. And make sure that students are aware that these really hot off the presses kind of advances exist. Because they can quite frankly, revolutionize businesses. Right.
I mean, there’s a, there’s a technique I work with called agent-based modeling, but a lot of times it’s used for exactly that purpose, right. Trying to understand pedestrian flow through a location, right.
And that’s something that we could do, but we don’t. You know, the part of the problem is that the tools are at one level, right.
And our knowledge of how to use them are at a different level right now, right, and we need to bring those two into closer balance with each other. And I think, you know, through a series, you know, revising our curriculum, working on our curriculum, kind of incorporating more and more than we’re doing a great job already, right?
The classes we’ve already got are fantastic in this space, you know, we’re never at BAI going to be developing the next generation computer algorithms or optimization tools or anything like that. That’s not our goal, but our goal is to take cutting edge state-of-the-art technologies and teach our students how to use it in applications that’s never been used on before.
Right. which is really understanding business problems. Right. and so that’s really what our goal at the BAI is.
Jenny: Well, I can’t thank you all enough for joining us today, but I can’t let you go without asking a fun question at the end, I’ve been asking our guests this. So, you know, we are a college and we have students in a range of 18 and on up. So I’d like you to think back for a moment when you were 21. If you could go back to your 21-year-old self and give yourself a piece of advice, knowing everything that you know now, what would you tell yourself?
Richard: I would probably tell myself to study a little more, but I think give yourself time to learn and grow in your twenties. you know, don’t be in a rush to define what your career is going to look like. There’s a lot of growth that happens in those twenties, and I think exposing yourself to as many opportunities as possible and as many people as possible different points of view, is really it’s, it’s important. And I think we probably force people into tracks too soon.
These skills that you’re building will be applicable in so many different ways that we can’t even predict. Don’t feel that your future is defined right now, but just build skill and build context and give yourself a few.
Erik: Yeah. So I think it’s a tough question. I think I’d probably two things. I think one, I mean, back to my comment earlier about curiosity and asking more questions, and then I think, you know, I liked Richard’s answer, just around patience. You think about you know, how long is your career and, and, you know, we, we get so focused on, on little pieces of it along the way. And I think you kind of, you’ve looked back on it and you’d say, you know, it’s okay. It would have been okay to maybe be a little bit more patient along the way.
Bill: I would definitely second both of those notions, right. I have a PhD in artificial intelligence and somehow, I’m now a professor of marketing. So I, I think I would look back and give myself permission to kind of wander a little bit. Right. To try and figure out what I really want to do. You know, the one other thing I would add that, you know, personally right now, given where I currently am, the thing that I would tell myself is take more statistics.
The last statistics class I took was an undergrad. Right. And, and hopefully my PhD students I’m advising and statistics, aren’t listening to this podcast right now because, you know, I mean, I I’ve had to self-educate myself a lot in that space. And I think having a few more courses in statistics would really help me, you know, not just be a better professor of business analytics, but also just be a better person. It helps you make better decisions. If you understand better how statistics works.
Richard: I, you know, I’ve worked with Erik so many years now and Erik is you know, he may not have a master’s degree in statistics, but he could probably ace the course. I can, I can see it in his eyes when I’m playing amateur statistician, and he just sort of smiles and, and I think, yeah, I really have no idea what I’m talking about. And I agree with you, statistics is important
Jenny: We call you fake it until you make it.
Richard: Or you can hire people like Erik.
Jenny: That’s awesome. Thank you, guys, so much again for your time today. It’s exciting to hear about the work that Home Depot is doing. And Richard, it’s always, always fun to have alumni back and hopefully you’ll stay engaged with us down the road too. And Erik, all the best as you continue to build out that data science forum and Bill, I know where you’re at, so we’ll make sure we check in with you often, but thank you. Thank you again, all of you for your time.
Erik: Jenny, thank you very much.
Richard: Thanks for having us. Go Pack.
Bill: Thanks for having us, as always.
About the Poole Podcast
The Poole Podcast is a think and do conversation about the relationship between academics and industry. Each episode will share research and ideas from inside the classroom from our incredible NC State faculty and explore how it’s being translated into practice. Released every two weeks on Monday.
- Categories: