Researchers Develop Framework for More Efficient Adoption of Medical Innovations
Poole College professor’s research shines light on the importance of integrating new diagnostic tests into existing clinical workflows.
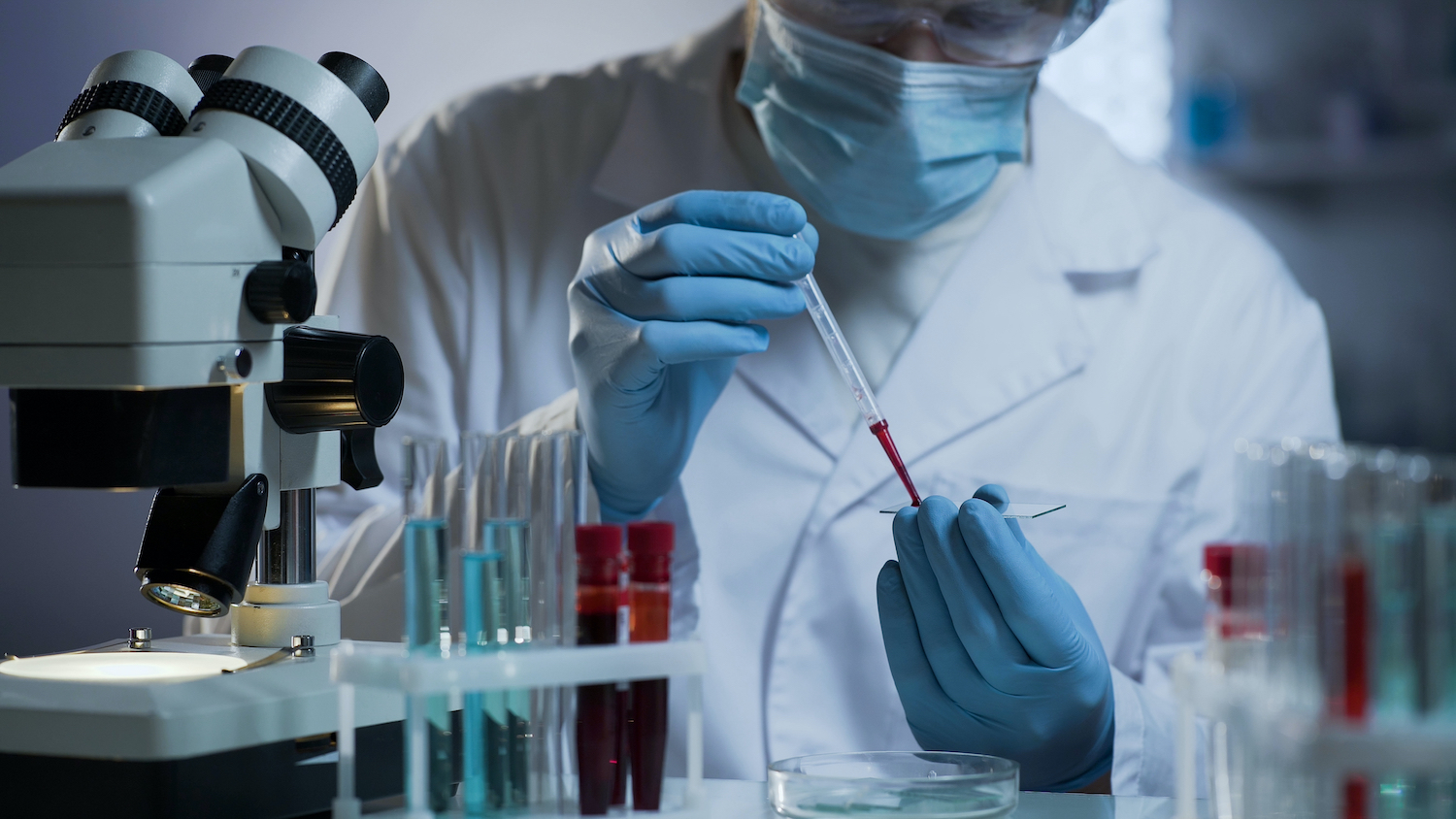
By Caroline Barnhill
Significant amounts of time, money and energy go into developing new, effective diagnostic tests – countless research hours, millions of dollars in equipment and dedicated laboratory space. But what happens when the medical providers elect not to use these new tools for reasons such as a perceived increase in their workload? It’s a question like this that led Alice M. Mitchell, MD., an emergency medicine doctor and associate professor of emergency medicine at the Indiana University School of Medicine, to approach a group of operational researchers, including Poole College’s Sebastian Heese.
Heese serves as a department head and Owens Distinguished Professor of Supply Chain Management at NC State’s Poole College of Management.
“When diagnostic tests are developed, researchers usually evaluate the sensitivity and specificity of the test – but not how those tests would be used within the existing workflows,” Heese explains.
The team’s research aims to bridge the gap between medical research and workflow management by not only considering the decisions behind adopting new diagnostic tests but also looking at how new tests are integrated into existing clinical workflows. To examine these issues, the researchers looked into a specific diagnostic test that screens for pulmonary embolism (PE).
Not only that, Dr. Mitchell explained how physicians are often reluctant to use additional testing if they see it as a workload burden.
“If a patient comes to the emergency department exhibiting symptoms such as chest pains or shortness of breath, they are suspected of having PE. At that point, they will be given a pretest survey that will be scored and those above a certain threshold will be sent to have a CT scan to confirm whether or not they actually have PE,” Heese says. “While CT imaging is extremely effective in detecting PE, it does come with its own set of risks – such as an increased risk of cancer. Additionally, CT machines are often one of the hospital’s most heavily utilized and expensive diagnostic resources.”
Mitchell, an emergency department (ED) physician, was interested in introducing a new diagnostic test called D-dimer – a fast, simple blood test that can be used to rule out patients who do not have PE. However, while the test has a high sensitivity, it also has a low specificity – meaning it could produce many false positives, which could lead to an excessive amount of follow-up testing – potentially leading to increased patient length-of-stay, staff workload and congestion within the emergency department. Mitchell was concerned that the number of false positives might prevent ED physicians from adopting the test, since they would not want patients to be unnecessarily routed to the CT for confirmation, resulting in double testing.
“Not only that, Dr. Mitchell explained how physicians are often reluctant to use additional testing if they see it as a workload burden,” Heese continues.
The research team developed an analytical framework for evaluating the impact of introducing a new diagnostic test into a busy hospital environment that considers both the clinical and operational impacts of the new test – capturing tradeoffs such as the fact that the test could lead to false positives, thus leading to repeated testing of patients, but could also be used to rule out the possibility of PE, thus reducing expensive and potentially harmful CT scans.
The framework we developed can be used by other hospitals – providing them with easily interpretable guidelines for clinical adoption decisions.
They found that conventional medical criteria can lead to poor decision-making in both research development and clinical practice. For example, a test with high sensitivity may be overvalued by researchers but rejected in practice due to operational inefficiencies. Moreover, they found criteria currently used for adoption decisions to ignore the operational impact of the new test and overestimate the new test’s system-level misdiagnosis rate, leading to unnecessary rejection of the new research. These criteria also fail to account for system-level implications of the new test – such as the overuse of existing diagnostic equipment when it might not be necessary.
“The framework we developed can be used by other hospitals – providing them with easily interpretable guidelines for clinical adoption decisions,” Heese says. “Moreover, it can guide medical research regarding which test characteristics to focus on to improve the likelihood the developed tests will be adopted into practice.”
The paper, “An Operational Framework for the Adoption and Integration of New Diagnostic Tests,” is published in Production and Operations Management. The paper was co-authored by Pengyi Shi of Purdue University, Jonathan E. Helm of Indiana University, and Alice M. Mitchell of the Indiana University School of Medicine.
The study abstract follows.
“An Operational Framework for the Adoption and Integration of New Diagnostic Tests”
Authors: Pengyi Shi, Purdue University; Jonathan E. Helm, Indiana University; H. Sebastian Heese, NC State University; and Alice M. Mitchell, Indiana University School of Medicine
Published: February 2021, Production and Operations Management
DOI: 10.1111/poms.13263
Abstract: The gap between medical research on diagnostic testing and clinical workflow can lead to rejection of valuable medical research in a busy clinical environment due to increased workloads, or rejection of medical research in the laboratory that may be valuable in practice due to a misunderstanding of the system-level benefits of the new test. This has implications for research organizations, diagnostic test manufacturers, and hospital managers among others. To bridge this gap, we develop a Markov decision process (MDP) from which we create “adoption regions” that specify the combination of test characteristics medical research must achieve for the test to be feasible for adoption in practice. To address the curse of dimensionality from patient risk stratification, we develop a decomposition algorithm along with structural properties
that shed light on which patients and when a new diagnostic test should be used. In a case study of a partner Emergency Department, we show that the conventional myopic medical criterion can lead to poor decision making in both research development and clinical practice. In particular, we find that specificity—long a secondary consideration and often overlooked in the research process—is, in fact, the key to effective implementation of new tests into clinical environments. This myopic approach can lead to overvaluing or undervaluing new medical research. This mismatch is accentuated when a simple (current) policy is used to integrate research into the clinical environment compared with our MDP’s policy—poor implementation of a new test can also lead to unnecessary rejection. Our framework provides easily interpretable guidelines for medical research development and clinical adoption decisions that can guide medical research as to which test characteristics to focus on to improve the chances of adoption.
- Categories:
- Series: